“Scale Your Marketing Insights, Not Your Infrastructure”: Lessons from High-Performance Marketing Teams
Marketing data isn’t just another data problem—it’s a constantly evolving challenge. Learn how high-performance teams scale insights, not infrastructure, by leveraging specialized solutions to simplify complexity and unlock actionable intelligence.
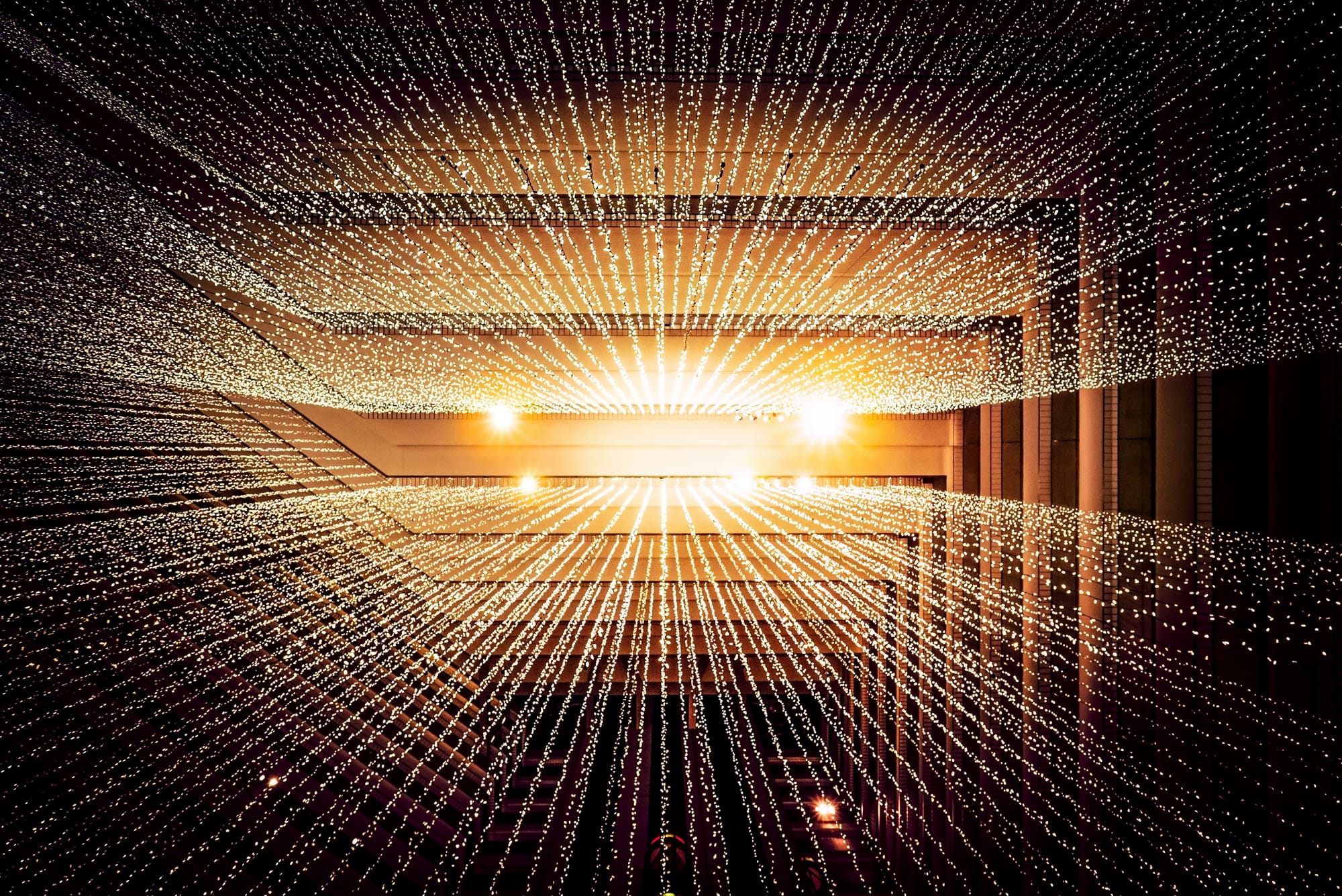
Somewhere deep in the data infrastructure of many large consumer companies is a team wrestling with an increasingly complex problem: how to effectively process, analyse and derive insights from marketing data at scale. At first glance, this might seem like a solved problem. After all, we live in an era of powerful off-the-shelf data tools. Everything from data warehouses like Snowflake to transformation tools like dbt to visualisation platforms like Looker.
But here at Clarisights, we don't think marketing data is just another data problem that can be solved by stitching together general-purpose solutions. To understand why this seemingly straightforward challenge is more complex than it first appears, Sidin Vadukut, sits down with Ashu Pachauri, CTO at Clarisights, to explore the unique characteristics of marketing data, and why traditional approaches often fall short.
Sidin: Ashu, what makes marketing data uniquely challenging, especially for large multi-geography, multi-product companies?
Ashu: The biggest challenge with marketing data is that it's a rapidly evolving space. Just look at the changes we've seen in the last couple of years - the (effective) deprecation of third-party cookies, the growing importance of incrementality analysis, and the push for better creative reporting. These changes create multiple dimensions of complexity.
First, there are constantly emerging ways of looking at and gathering this data. You can't just put a system in place and forget about it. You have to keep pace. This means constantly maintaining and evolving your data infrastructure. Take the iOS 14.5 update, for instance. When Apple introduced App Tracking Transparency, it fundamentally changed how marketers could track and attribute user behaviour. Suddenly, existing data pipelines and attribution models needed complete revamps.
Second, there's the challenge of scale. We're talking about millions of rows of data daily for larger companies. A typical e-commerce company running campaigns across multiple geographies might be ingesting data from Google Ads, Meta, TikTok, display networks, web analytics, app analytics, and CRM systems. Each source might generate hundreds of thousands of rows daily, and that's before you start joining these datasets together for cross-channel analysis.
Sidin: Can you give us a sense of what this scale looks like in practice?
Ashu: Let's use a concrete example. Imagine a company running digital marketing campaigns across 20 countries. They advertise on Google Ads, Facebook, and TikTok, with hundreds of campaigns running simultaneously. Each campaign might have dozens of ad groups, each ad group multiple ads, and each ad generates performance data across 30-40 metrics every hour.
Just for Google Ads alone, you're looking at: 20 countries × 100 campaigns × 10 ad groups × 5 ads × 35 metrics × 24 hourly data points = over 84 million data points daily. And that's just one channel. Now multiply this across all your marketing channels, then add the complexity of joining this with conversion data from your web analytics and CRM systems.
Sidin: I imagine many companies might be tempted to think, "We have these wonderful off-the-shelf products - Snowflake, dbt, Fivetran - surely we can just stitch these together to solve our problem?"
Ashu: That's exactly where the journey typically starts, and it's the natural assumption. Companies usually already have an internal data team and some way to access part of this data for other purposes. So they think, "Let's just add a metadata layer and visualisation capability on top of our existing warehouse." It seems like a straightforward project - maybe a couple of months with two people.
Let's walk through how this typically plays out. The team starts by setting up data pipelines using something like Fivetran to pull data from various marketing platforms into their Snowflake warehouse. They use dbt for transformations and maybe Looker for visualisation. Initially, it works - you can create some basic dashboards showing spend, impressions, and clicks across channels.
Sidin: What happens next?
Ashu: Problems emerge once marketers actually start using the system. Let's say a marketing team wants to analyse their customer acquisition cost (CAC) by customer segment and marketing channel. Seems simple enough, right? But here's where it gets complicated.
First, you need to join ad spend data with conversion data, but these often live in different systems with different granularity. Your ad platforms might report spend at the ad level, while your CRM tracks conversions at the user level. You need to build complex transformation logic to make these datasets compatible.
Then the marketing team realises that they might need to exclude certain types of spend from their CAC calculations - maybe promotional campaigns or brand awareness campaigns. Now they need a way to tag and categorise campaigns, which means either modifying your data pipeline or creating new tables and joins.
And this is just the beginning. Soon they want to create custom metrics for search campaigns. But this metric only makes sense for certain channels, so you need conditional logic in your transformations.
Sidin: So, companies might then look to add more tools to solve these emerging problems?
Ashu: Exactly. The natural next step is to buy even more products like Funnel to handle data ingestion. Again, it seems like an easy choice initially. But let's break down the costs:
For a company processing our earlier example volume - about 100 million rows daily across channels - you might be looking at:
- $10,000-15,000 monthly for data ingestion tools
- $20,000-30,000 monthly for your data warehouse compute and storage
- $5,000-10,000 for transformation tools and scheduling
- $10,000-20,000 for visualisation platforms
- Plus the cost of at least 2-3 full-time engineers to maintain this stack
And these costs keep growing. Every time marketers need a new data source or want to track new metrics, you're adding to your ingestion costs. Every new analysis or dashboard increases your warehouse compute costs.
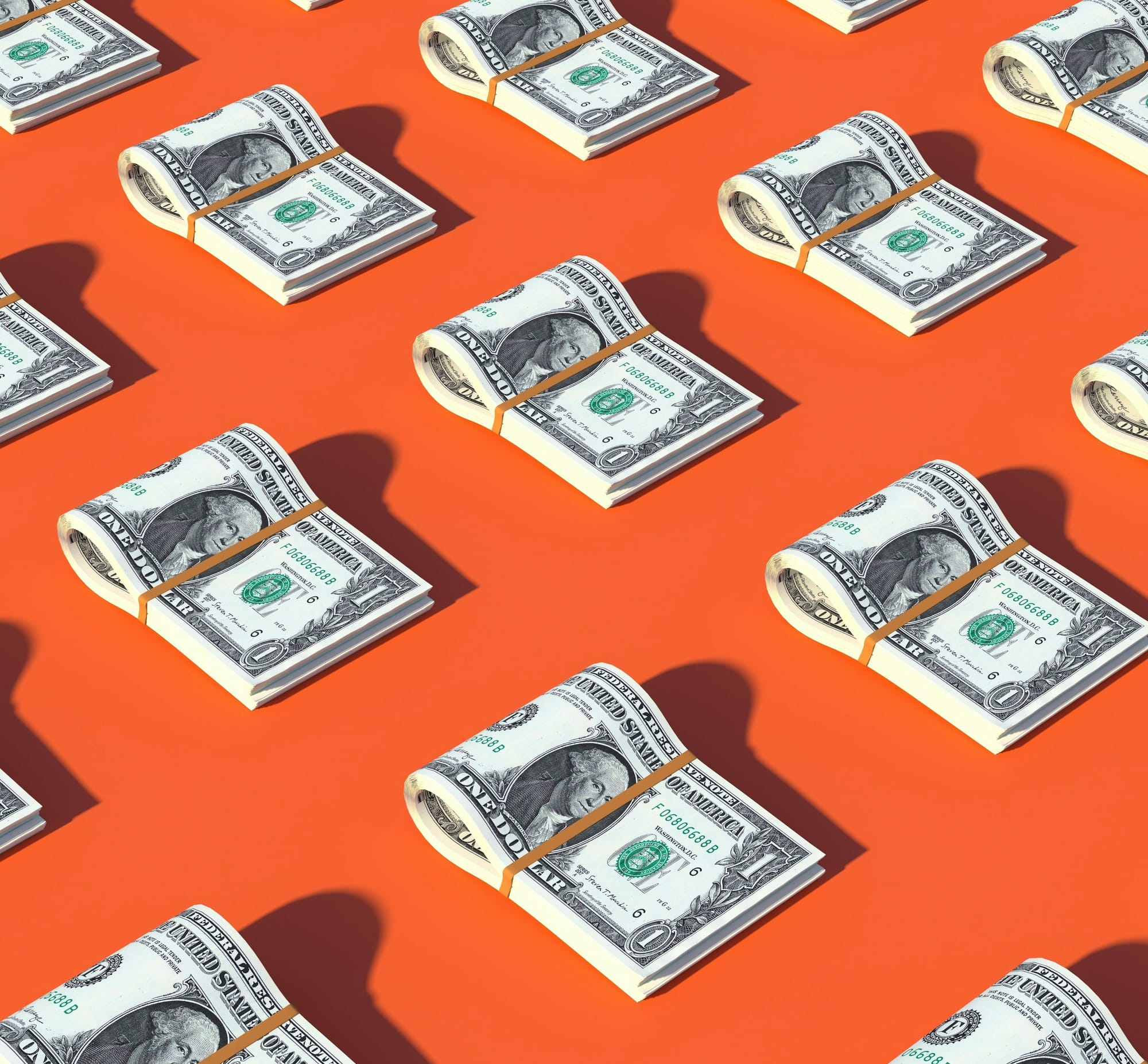
Sidin: What happens when teams try to optimise these costs?
Ashu: Teams might try to reduce costs by limiting data refresh rates or aggregating data more aggressively. But this creates new problems. Maybe your daily reports now only update once every 6 hours to save on compute costs. Suddenly, marketers can't react quickly to campaign performance issues.
Or you decide to pre-aggregate data to reduce query costs. But then marketers need to analyse data at a granularity you didn't anticipate - maybe they want to look at performance by creative element or audience segment. Now you need to modify your entire data model.
Sidin: One interesting point you made earlier is that people often think of this as just a read problem - ingest data, create dashboards, done. But that's not really how marketers work with data, is it?
Ashu: That's a crucial point. Modern marketing teams don't just consume data - they need to constantly transform and enrich it.
Take campaign tagging. Marketers need to categorise their campaigns by objective, funnel stage, target audience, and more. This isn't just labelling - it requires the ability to write these classifications back into the data itself. Similarly with budget allocation: Teams are constantly recording and adjusting planned versus actual spend, which means writing budget data that needs to be seamlessly joined with actual performance data.
Modern marketing teams don’t just consume data – they need to constantly transform and enrich it.
Then there's the complexity of custom metrics. Marketing teams need to create calculated metrics that combine data from multiple sources, and these aren't just simple ratios. They often involve conditional logic, time-based calculations, and cross-channel components. And let's not forget about data corrections - sometimes platform data is simply wrong and needs adjustment. Maybe Facebook reported some conversions incorrectly, or Google Ads double-counted some clicks. Marketers need to be able to fix these issues directly.
What makes this particularly challenging is that each of these write operations needs to be tracked, versioned, and made available in a way that doesn't break existing reports or analyses.
Sidin: And that's the case for why a specialised vertical SaaS solution might make more sense.
Ashu: Yes. It comes down to unit economics and optimisation opportunities. When you're building with off-the-shelf tools, you're paying margins at every layer of the stack. But more importantly, you're using tools that are optimised for general purposes, not specifically for marketing data, and not necessarily because they work well with each other.
For example, in a general-purpose data warehouse, calculating year-over-year growth for your campaign metrics becomes a complex operation. You need to join fact tables with date dimensions, create window functions for historical data, calculate growth rates, and then store these results for quick access. This process might require scanning terabytes of data and cost hundreds of dollars in compute costs. But with a specialised solution, you can optimise specifically for these types of calculations, storing data in ways that make these comparisons efficient and implementing caching strategies that make sense for marketing use cases.
The same principle applies to data ingestion. General ETL tools treat all data sources equally, but marketing data has specific patterns - daily aggregates, hourly performance metrics, and real-time budget pacing. By optimising for these patterns, you can significantly reduce both cost and complexity.
Sidin: So, what's the alternative to building this internal stack?
Ashu: The alternative is using a specialised platform built specifically for marketing analytics. This is actually a deep topic that deserves its own discussion, but let me give you the key points.
A specialised platform provides a data model that inherently understands marketing concepts - the relationships between campaigns, ad groups, and conversions across platforms. It handles common marketing scenarios like budget pacing, cross-channel attribution, and creative performance analysis out of the box. Most importantly, it's optimised for how marketers actually work with data, from comparing performance across time periods to blending data from multiple channels.
So, for example, something as basic as calculating ROAS across channels becomes dramatically simpler. Instead of pulling cost data from multiple platforms, joining with conversion data, and managing attribution windows across different systems, it's handled seamlessly in one place. This integration doesn't just reduce complexity - it makes the entire process more efficient and cost-effective.
The end result is that marketers get the capabilities they need without managing multiple tools, while companies get better performance at a lower cost. It's a classic example of how vertical specialisation creates value that wouldn't be possible with general-purpose tools.
Sidin: Let's talk about the human cost of building an in-house solution. What kind of team would a company need to put together?
Ashu: This is often a hidden cost that companies don't fully account for in their initial planning. To build and maintain a robust marketing analytics stack, you typically need a robust staff. You'll need 2-3 Data Engineers to build and maintain data pipelines, optimise warehouse performance, handle schema changes from marketing platforms, and maintain complex transformation logic.
The Backend Engineering team needs to be similarly sized. They'll be responsible for building APIs for data access, implementing custom metric calculations, handling user authentication and access control, and maintaining metadata services.
Frontend engineers (1-2) will focus on building visualisation interfaces, implementing interactive features, and maintaining dashboard performance as data volumes grow.
A DevOps/SRE team (1-2) manages infrastructure, monitors system performance, handles scaling and optimisation, and maintains security compliance.
Your Analytics Engineers will build and maintain data models, implement business logic, ensure data quality, and support the constant stream of requests from the marketing team.
And this is just the technical team. You also need product managers, technical project managers, and often dedicated support staff. We're talking about a team of at least 10-12 people, which in most markets means a fully-loaded cost of $1.5-2 million annually.
Sidin: And this team needs to be maintained continuously...
Ashu: Exactly. This isn't a "build once and maintain" situation. Marketing data requirements evolve constantly. Every time Facebook changes its API, or Google introduces a new campaign type, or Apple updates its privacy requirements, this team needs to update the stack.
You also have to factor in the operational challenges. When key team members leave, you don't just lose their labour - you lose crucial institutional knowledge about how your custom stack works. Training replacements takes time, during which your marketing team's effectiveness might be impacted.
Sidin: So, what's your advice to performance marketing teams considering their options?
Ashu: Teams need to be pragmatic about their choices. Building your own stack might seem attractive, especially for engineering-driven organisations. You might think you'll have more control and flexibility. And in theory, you do.
But in practice, you're signing up for a massive ongoing commitment that isn't core to your business.
Every engineer working on your marketing data stack is an engineer not working on your product. Every dollar spent maintaining infrastructure is a dollar not spent on actual marketing.
Think about how companies today use cloud providers instead of building their own data centres. Yes, running your own data centre gives you more control, but for most companies, it's simply not worth the cost and complexity. The same principle applies to marketing analytics infrastructure.
The pragmatic approach is to leverage specialised solutions that have already solved these complex problems. This doesn't mean giving up control - it means being strategic about where you invest your resources. The marketing technology landscape will continue to evolve, privacy requirements will keep changing, and the volume of data will keep growing. The real question isn't about building for today's challenges - it's about maintaining and evolving solutions for tomorrow while keeping costs under control.
That's the calculation teams need to make: weighing not just the initial build cost, but the total cost of ownership, including the opportunity cost of diverting engineering resources from core business value.
The real question isn't about building for today's challenges - it's about maintaining and evolving solutions for tomorrow while keeping costs under control.
Sidin: This brings up an interesting point about competitive advantage. Some might argue that building your own stack gives you an edge over competitors.
Ashu: This is a huge misconception that needs addressing.
When you're operating as a high-performance marketing organisation, your competitive advantage doesn't come from the infrastructure you've built to process data - it comes from how you use that data to make better decisions.
Think about the most successful performance marketing teams we work with. Their edge comes from their ability to spot trends quickly, to understand their audience deeply, to optimise campaigns creatively, and to allocate budgets intelligently. None of these advantages come from having built their own data pipelines.
If anything, building your own stack can be a competitive disadvantage because it diverts focus from what actually matters. The time your team spends maintaining infrastructure is time they're not spending analysing customer behaviour or identifying new opportunities.
Your competitive advantage doesn’t come from the infrastructure you’ve built to process data – it comes from how you use that data to make better decisions.
This is why many of our most sophisticated customers have stayed with us for years, despite having the resources to build their own solutions. The companies that consistently outperform their competitors are the ones that focus their energy on deriving insights and taking action, not on maintaining infrastructure.
If you are a Hyperscale company ready for real insight into your data, contact us!